CAD is prior knowledge, what can we do with it?
Design engineers make multiple decisions on everyday company activities. Those decisions have ripple effects at all levels of company operations. Harnessing and analysing all the data holistically can enable more efficient processes and ultimately assist decision-making. Here, Artificial Intelligence (AI) could help combine prior knowledge with novel product ideas intelligently to exploit their relationships fully. In the long term, AI agents will provide recommendations to enhance the CAD engineer’s cognitive ability to automatically generate an array of design alternatives. An example of productivity enhancement by AI has been recently developed within Vertex G4 mechanical design engineering software.
November 2021
Iñigo Flores Ituarte
Doctor of Science (Technology)
Professor and Docent at Tampere University
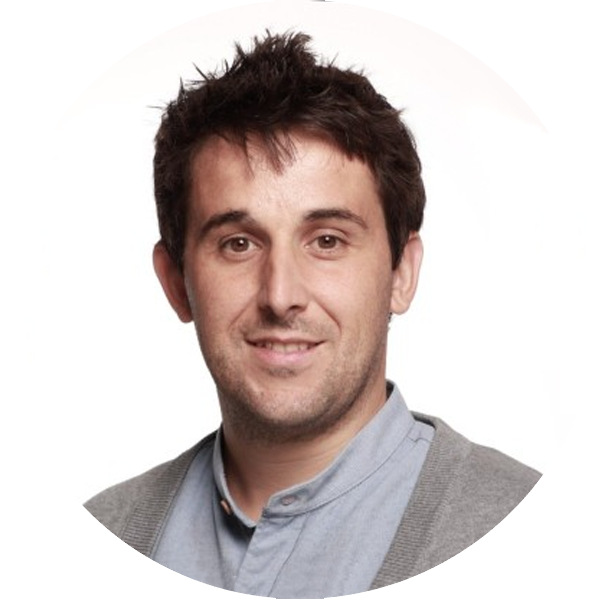
The negative impact of bad design increases as one goes through the development cycle of a product. At the same time, the likelihood of conceiving a faulty design is much higher at the initial stages of the development process, where information and domain knowledge is often partial, ambiguous, or entirely lacking. Design engineers make multiple decisions on everyday company activities. Those decisions have ripple effects at all levels of company operations, from manufacturing to supply chain and beyond. New computer-aided expert systems will allow engineers to significantly improve the product development process with a life cycle perspective.
The questions are: How can we find as many faulty designs as possible and dismiss design alternatives that lead to unwanted results? How can we assist design engineers in their work to help them reduce development time? How can we increase the likelihood of generating perfect design solutions with “zero-waste” from design to verification, and manufacturing? How can we still explore enough design alternatives at the initial stages, that are feasible and optimal considering all the product life cycle stages?
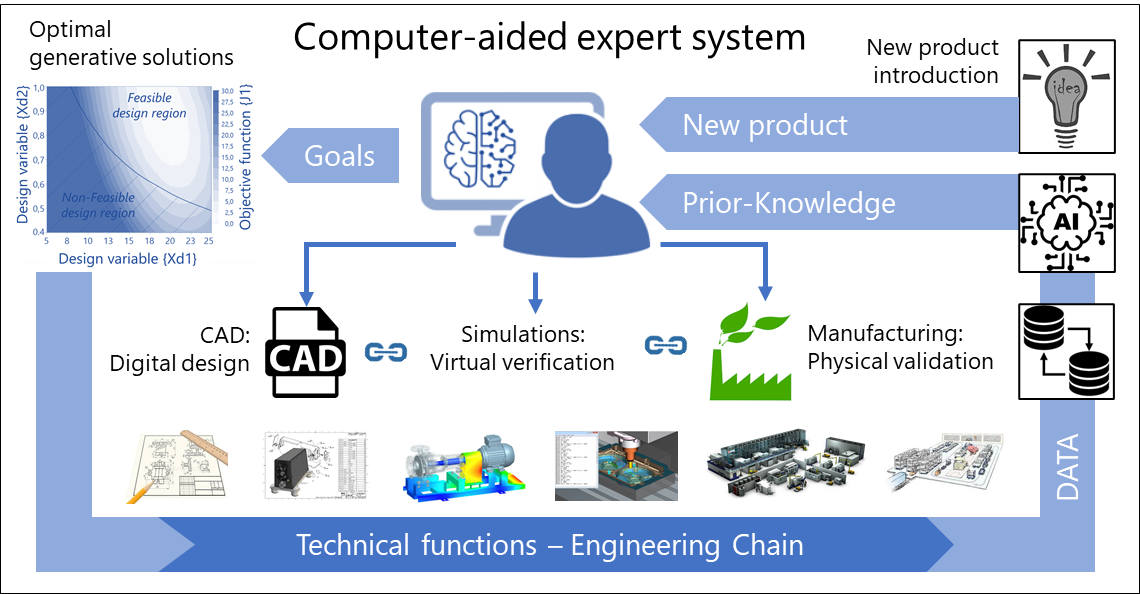
Artificial intelligence enhancing CAD processes
Complex product development is driven by the interaction of CAD and simulation methods with virtual verification and physical validation. The technical functions of the engineering design chain become a rich source of data. Data is often unstructured and spread across the entire product development process. Thus, including design concepts, sketches, CAD, simulations, test experiments, intertwined product life cycle management data, and manufacturing process-related data. Hypothetically, harnessing and analysing all the data holistically can enable more efficient processes and ultimately assist decision-making that (i) leads to reduced development time in CAD processes, virtual verification, and physical validation (ii) dismiss early enough design alternatives that lead to unwanted results, and (iii) explore design alternatives that are feasible and optimal considering all the stages of the product life cycle.
However, companies are not equipped with the tools to exploit the correlations between different data sources or utilize prior knowledge effectively to assist product development and decision making in an automated manner. Engineering companies are not to blame. Instead, we should focus on developing expert computer-aided systems that enhance humans’ processes from CAD to manufacturing. Exploring the relationships between different data sources could become extremely valuable. Here, Artificial Intelligence (AI) could help combine prior knowledge with novel product ideas intelligently to exploit their relationships fully.
Engineering design is data, data is prior knowledge
Every design alternative requires its computer representation. In the traditional realm, the designer would try out a limited amount of iterative design rounds using his intuition and tacit knowledge to analyse each concept. This implies that design engineering becomes a highly manual process and requires knowledge from multiple domains. Sketching is at the heart of every engineering Computer-Aided Design (CAD) software. Multiple 2D sketches are turned into solid models that are assembled for virtual verification. In the past, before CAD was a thing, most products were developed by a trial-and-error approach. Prototypes were fundamental in the development process, and they were built iteratively until product ideas reached the required maturity.
Nowadays, CAD modelling has replaced physical verification to a considerable extent, and engineers can check virtually for part interferences in complex product assemblies. Thus, making sure that product parts will fit as planned after manufacturing. In turn, new product development requires multiple CAD designers to iterate a vast amount of design alternatives. Each design iteration requires hours and hours of often repetitive and slow tasks. Sketching, constraining the sketches, making relationships between geometrical parameters, creating surface models or solid models, and ultimately testing the models into complete assemblies takes considerable resources.
Engineering creativity is often limited by how fast we can iterate and generate new virtual designs and assembly alternatives. Besides, the engineers need to communicate and exchange product-related data across engineering teams at different geographical locations to link part-procurement and the bill of materials with the manufacturing and supply chain. Product data management systems (PDM), or life cycle management systems (PLM), are the ultimate result of the technical functions within the engineering chain. It encodes the product-related knowledge as digital data and serves the company controlling functions, economic planning, and supply chain.
However, it is essential to understand that AI cannot entirely replace engineering companies’ technical functions including CAD, simulation, and verification processes. Instead, in the short term, AI agents will assist engineers in making quicker and better decisions during CAD, reducing development time. AI has become a powerful tool to automate repetitive and routine tasks. In the long term, AI agents will provide recommendations to enhance the CAD engineer’s cognitive ability to automatically generate an array of design alternatives while evaluating multiple optimization objectives with a life cycle perspective.
A descriptive example of productivity enhancement by AI has been recently developed within Vertex G4 mechanical design engineering software. The new AI-based system in Vertex G4 can automatically select assembly constraints based on geometrical features and available degrees-of-freedom. The AI-agent has studied how Vertex’s customers use assembly constraints in their design processes (i.e., gathered prior-knowledge), and uses that data as the basis for decision making. In testing, the system selected the same constraint as professional CAD designers in around 95% of tested cases. The system is a concrete example of the potential of AI in aiding CAD users by decreasing the required design time and enhancing the CAD user experience. In the future, the system will be able to learn and adapt to match customer specific workflows and advance the assembly constraint adding process even further. If interested, read a more in-depth description of how the system works at thesis “Automating CAD assembly constraints using supervised deep learning”.
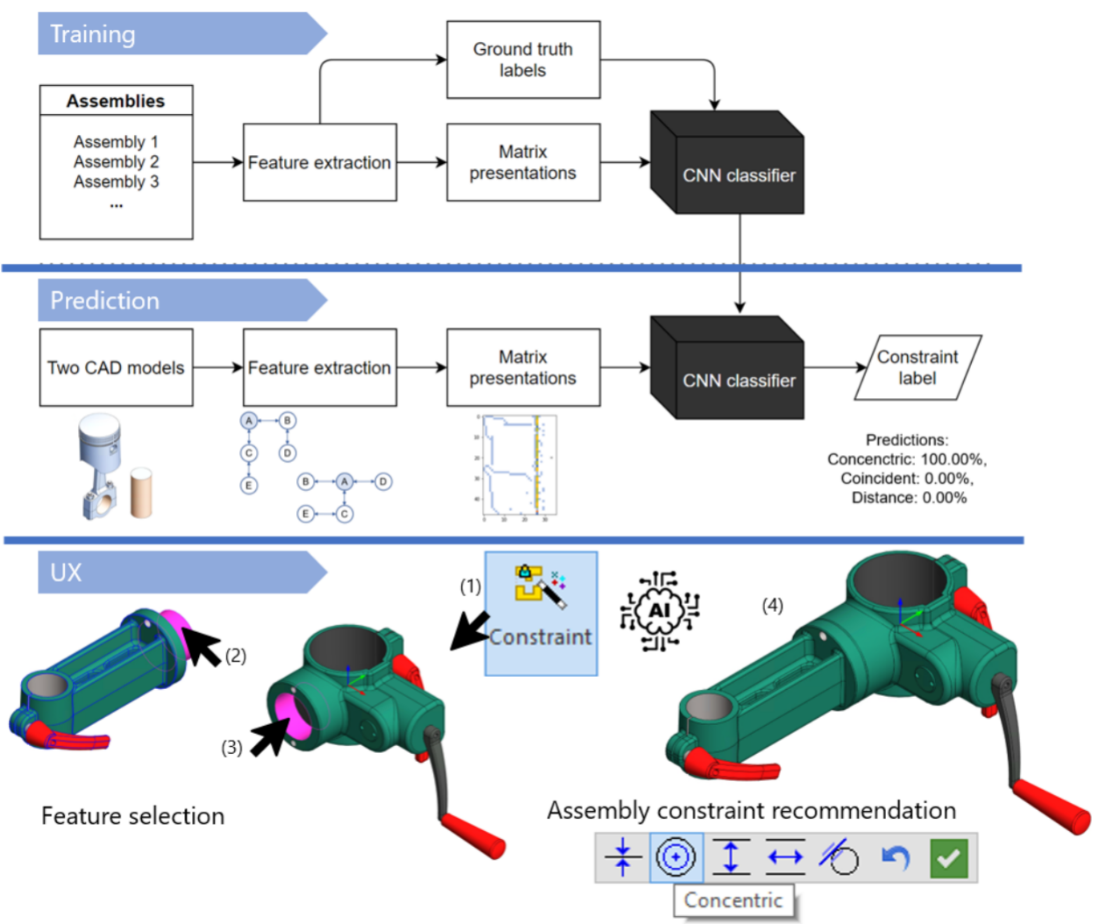
A product life-cycle perspective: Can we embed sustainability early on?
Increases in efficiency by digital technologies will help us reduce energy and materials usage and environmental footprint. Manufacturing activity is responsible for 54% of the world’s energy consumption and 20% of global CO2 emissions. The future for sustainable production requires that design engineering, manufacturing systems, and supply-chains are better connected, intelligent, and eventually “cognitive”. A promising solution to sustainability lies at the interfaces between advanced engineering design software, digital manufacturing technologies, and AI.
When we transform this idea to the processes withing the engineering chain, we could envision how a computer-aided expert system will complement humans with a conscious intellectual activity that involves prior-knowledge, thinking, and helping to figure the best solutions. Let us imagine computer-aided expert systems working together with us to help us simplify and eliminate repetitive task as well as to generate product layouts with different structures and configurations. All we need to focus on is selecting the most appealing alternatives. The concept behind generative design brings optimization elements to AI-agents which could work to enrich the engineering chain with CAD, simulations, and PLM systems. Generative design is not only a modern way to create futuristic parts, and instead we need to understand its full potential. For example, to generate multiple layouts of a manufacturing plant to focus more on choosing the optimum configuration based on a set of constraints and tackle multiple optimization objectives simultaneously.
The hypothesis is that current advances in AI combined with digital design and manufacturing can bring us into the next generation of computer-aided software systems that assist a decision-maker in exploring trade-offs among objectives to help us make improved decisions. To sum it up, the future of computer-aided systems is to assist streamlining unproductive tasks and remove “waste” across the entire engineering chain. For example, to help us conceive feasible compositions of materials, geometrical designs, machine topologies, manufacturing instructions, manufacturing layouts, and supply-chain configurations while being capable of evaluating in real-time the impact of our ideas at system performance level to optimize the trade-offs between economic implications, development time, material requirements, energy consumption, and environmental footprint.
Iñigo Flores Ituarte is a Doctor of Science (Technology) graduated from Aalto University. His background is in mechanical engineering with industry experience in several companies, including Konecranes and Nokia. Currently, he works as Industry Professor and Docent at Tampere University. His area of research is on Digital Design and Manufacturing, with skills in engineering design and manufacturing trends. He specializes in digital design, digital manufacturing, CAx, simulation, predictive modelling, data science, optimization, and advanced manufacturing processes.
Vertex Systems is a Finnish provider of CAD and PLM/PDM software solutions. With Vertex software, you speed up design, reduce design errors and improve productivity. Check out the Vertex software.
Vertex Systems on suomalainen suunnittelun ja tiedonhallinnan ohjelmistoratkaisujen toimittaja. Vertex-ohjelmistojen avulla nopeutat suunnittelua, vähennät suunnitteluvirheitä ja parannat tuottavuutta. Tutustu Vertex ohjelmistoihin.